Short Courses
If a short course needs to be canceled due to a lack of registrations, we will inform you and refund the registration fees.
- Introduction to the analysis of compositional data
- Compositional Statistics for Geochemistry
- Geoscientific data analysis and mineral prospectivity mapping using open-source geospatial applications (GisSOM and QGIS) - cancelled
- From data preprocessing to precision: best data management practices for data-driven modeling in geoengineering
- Applied Geochemistry and Analytics - cancelled
- Round geology, square geostatistics, and how to make them fit - cancelled
Introduction to the analysis of compositional data↑
|
![]() |
![]() |
click here to get more information
Course description
Compositional data are vectors that show the relative importance of the parts of a whole. Typical examples are data in mol/liter, percentages, ppm, ppb, or similar, common in many fields of science, particularly in the geosciences. The classical statistical analysis of this type of data suffers from multiple problems, among them the one of spurious correlation. As a solution to these problems, J. Aitchison introduced the logratio approach in the 1980s. Since then, progress has been made in understanding the geometry of the sample space, the simplex of D parts.
Course objectives and learning outcomes
The course aims to introduce attendees to the principles and basic methods of compositional data analysis; how to apply them with Codapack; and how to interpret the results obtained. The course combines theoretical classes with practical data analysis.
Content
- The Aitchison geometry in the simplex and coordinate representation (2 hours).
- Exploratory analysis with CoDaPack: variation matrix, biplot and coordinates (2 hours).
- The CoDa-dendrogram. Orthonormal coordinates (ilr-olr) with CoDaPack.(1.5 hours).
- Compositional statistics. Regression. (1.5 hours).
Free software
- CoDaPack 2.02.04 downloadable from www.compositionaldata.com
- Compositions (in R)
- rob-Compositions (in R)
- zCompositions (in R)
- R Project for Statistical Computing: www.r-project.org
Compositional Statistics for Geochemistry↑
|
![]() |
![]() |
click here to get more information
Content
Geochemical data usually consist of either element concentrations or isotope ratios. For this type of data, their inherent properties limit the application of conventional statistical methods. There are two coexisting approaches to analyzing such data: Classical geochemistry has developed individual graphs and normalization rules for each type of problem to minimize common artifacts, but so far lacks general-purpose tools that would find wide application in geochemistry. Modern compositional data analysis, on the other hand, offers comprehensive and general solutions for the mathematically correct analysis of concentration and ratio data, but has so far failed to provide methods for many typical tasks and challenges in geochemistry. This course bridges the gap between the two approaches and provides compositionally coherent statistical methods for geochemistry. The discrepancy is addressed in three ways: - We learn which classical geochemical tools are already compositionally coherent, why they are coherent, and how to incorporate them into a fully compositional data analysis. - We learn how certain compositionally incoherent methods can be replaced by coherent methods for the same questions and how the two classes of methods are related. - We use an interactive class structure where participants are strongly encouraged to bring their typical methods and challenges, and we search for artifacts and construct bridging solutions together.
Who should take the course?
The course is intended for geoscientists who are interested in or have experience with statistical analysis of geochemical data. For individuals who have no prior experience with compositional data analysis, we strongly recommend combining this short course with the pre-conference short course "Introduction to Compositional Data Analysis".
Free software
- R Project for Statistical Computing: www.r-project.org
- Compositions (in R)
From data preprocessing to precision: best data management practices for data-driven modeling in geoengineering↑
|
![]() |
click here to get more information
Course concept
Advances in engineering equipment that can deliver massive in-situ data at runtime open the possibility to employ data analysis and data-driven modeling to ensure proactive risk management and enhance process optimization in geoengineering. However, obtained multivariate observational site-specific datasets are often incomplete and potentially corrupted and therefore require special techniques to be applied during the preprocessing step to ensure high-quality results from data analysis and data-driven modeling. The course will elaborate on methods and techniques for data quality checks, preprocessing, integration, and feature engineering.
This course is designed for audiences with a major in civil engineering and/or geosciences interested in data mining and does not require any particular expertise in programming. The course aims to show how the accuracy of data analysis depends on selecting the correct preprocessing strategy and will illustrate the applicability and limitations of main preprocessing steps using relevant examples from geoengineering and geotechnics. The real datasets (e.g., data from cone penetration test or tunnel boring machine) will be used throughout the course, bridging the gap between theoretical concepts and their applications.
Course objectives
To merge data science and civil engineering by- demonstrating the importance of data quality assurance and data preprocessing for the success of data-driven modeling
- explaining the limitations of analytical methods in geo-datasets
- showing how various techniques can be used to overcome the limitations and improve the precision of modeling
Learning outcomes
Audience will- review the knowledge discovery, data management, and the data life-cycle concepts
- refresh the concepts of data sparsity and dimensionality
- familiarize themselves with modern representation and formats for data storage
- understand the importance of data quality assessment
- learn the limitations of analytical methods in data-driven modeling when applied to geotechnical data
- get “ready-to-apply” solutions for overcoming analytical methods’ limitations with data preprocessing and data engineering techniques
- understand how the decisions made during data acquisition and processing affect the accuracy of data-driven modeling.
Course content
- Introduction to Data Science. Why do we care: errors, accuracy, and decision support.
- Structures and data types, with practical examples from geo-datasets. Data quality, storage, integration, and security. Data labeling and perception in labels.
- Data formats, precision, and structures. Data sparsity.
- Statistical and mathematical foundations of Data Science. Data-driven modeling: concepts, methods, applicability, and limitations. Machine learning: concepts, algorithms, limitations. Examples from geoengineering.
- Data preprocessing and feature engineering. Evaluation and validation metrics in Data Science. Data integration, rebalancing, and dimension reduction. Synthetic data: pros and cons.
- Sample workflows for quality assurance, preprocessing, and accuracy check for regression and classification tasks in geoengineering. Correlational analysis in preprocessing.
IAMG2023 sponsored by:
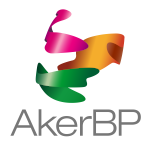
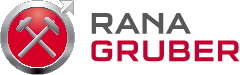
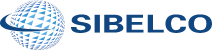