Keynote speakers and IAMG Awardees
Keynote Speakers
IAMG Awardees
- John Carranza - William Christian Krumbein Medal 2022
- Philippe Renard - John Cedric Griffiths Teaching Award 2022
- Qiuming Cheng - Distinguished Lecturer 2022
Keynote Speakers↑
Bruno Lévy↑
Bruno Lévy is the director of the Inria Nancy Grand-Est research
center, one of the eight research centers of Inria, the French research
institute for research in digital science and technology.
He defended his Ph.D. on computational topology and its applications
to subsurface modeling at INPL in 1999, then did a post-doc at
Stanford, then joined Inria in 2000.
From 2000 to 2015 he worked on surface parameterization,
mesh generation and optimal sampling. In addition to computer graphics,
Bruno's work has impacted the area of meshing and simulation of complex
geological structures. Since 2015, he has been working
on computational physics, and more specifically on applications of
optimal transport to fluid dynamics and cosmology.
Talk title: Computational optimal transport
I will give an introduction to optimal transport, a mathematical theory that makes it possible to measure distances between functions (or distances between more general objects), to interpolate between objects or to enforce mass/volume conservation in certain computational physics simulations. Optimal transport is a rich scientific domain, with active research communities, both on its theoretical aspects and on more applicative considerations, such as geometry processing and machine learning. I will summarize the main principles behind the theory of optimal transport, introduce the different involved notions, and more importantly, how they relate, with the aim of giving an intuition of the elegant theory that structures them. Then we will consider a specific setting, called semi-discrete, where a continuous function is transported to a discrete sum of Dirac masses. Studying this specific setting naturally leads to an efficient computational algorithm, that uses classical notions of computational geometry, such as a generalization of Voronoi diagrams called Laguerre diagrams.
Malcolm Sambridge↑
Malcolm Sambridge is a Professor at the Research School of Earth Sciences,
Australian National University (ANU) and works in the areas of
Seismology and Mathematical Geophysics.
He obtained a Certificate for Advanced Study in Mathematics from
Cambridge University in 1984, and a PhD in Geophysics from ANU in 1989. He
worked at the Carnegie Institution in Whashington, at the University of
Cambridge and at Caltech's Seismological Lab.
He published over 150 peer-reviewed papers covering data inference methods,
inverse theory, computational seismology, wave propagation,
earthquakes and earth structure, mathematical methods,
statistical inference applied to the Earth Sciences,
Monte Carlo methods and optimization.
In the area of Mathematical Geosciences, he made significant
contributions the application of Voronoi diagrams
to solve geophysical and geodynamic problems. Most importantly,
the cornerstone of Malcom's research has been
stochastic Bayesian methods for solving inverse problems in the
geosciences. One can cite in particular the 'neighborhood algorithm',
which he introduced to solve inverse problems based solely on
proximity information. More recently, he focused on transdimensional
inversion, where the number of model parameters is unknown.
Among other scientific awards and recognitions, Malcolm is a Fellow and Vice
President of the Australian Academy of Science, and a fellow of the
American Geophysical Union (AGU).
Talk title: Optimal transport and Geophysical inversion
The field of optimal transport is thought to have originated in the 19th century, when legend has it that Napoleon asked Gaspard Monge to rearrange his sand castles. That started a 200 year story of discovery and re-discovery of the mathematics of how to map, or transport, one curve (or probability distribution on to another). Leonid Kantorovich reformulated Monge’s problem in terms of more familiar linear programming which contributed to his winning the 1975 Nobel prize for economics. Cedric Villani pioneered the modern mathematical treatment of the topic and was awarded the 2010 Fields medal.
What has all of this got to do with Geophysics? Here exploration geophysicists have led the way and shown how to exploit OT in Full seismic waveform inversion. It turns out that optimal transport may be used as an alternate to Least squares measures to create a new type of data misfit function. It has been demonstrated that it has significant potential in nonlinear inversion by reducing the presence of local minima in misfit functions which would otherwise by highly multi-modal. Over the past decade this has created a flurry of excitement and activity in Seismic Waveform inversion in exploration geophysics, and a gradual appreciation of the topic more broadly. This talk will introduce OT for geophysical inversion in a more general context, and also discuss some new ideas and open questions which, as always, take the form of how do we best exploit these `pure’ mathematical concepts in an effective manner for practical outcomes.
Colin Daly↑
Colin did a degree in mathematics at Trinity College Dublin
and a PhD in Geostatistics at the Ecole des Mines de Paris.
He joined BP Research working on algorithmic development and applying
the methods in field studies across most of the basins in which BP
operated at the time. As the subject matured the inevitable division
of labour between practitioners and developers deepened in most companies.
He opted to go with the development side leaving BP to work
initially with Roxar and then with Schlumberger where he initially
worked on commercial software, particularly with the Petrel platform.
Contact with the range of software users and the surprisingly
wide variety of ways that they can use an algorithm has led
to him taking an interest in robust design particularly as tools
from Machine Learning become available for spatial probability models.
Talk title: Interpolations, realizations and scenarios - using ML spatially without big data
A typical geostatistical problem might only have sparse observations of the target variable, sometimes accompanied by (a possibly large) number of densely observed secondary data variables. The geostatistical approach constructs a spatial model using a random function (RF), with hypotheses such as stationarity, and uses this model to make predictions. As well as predictions, realizations of the RF are used to understand ‘typical’ behaviour of the target variable, often as Monte Carlo samples for further spatial nonlinear analysis, such as fluid flow. When there is too much uncertainty in the geoscience to focus on a single type of RF, scenarios are constructed by varying model type or significant parameters to cover the meaningful uncertainty. Some problems are that the RF models are difficult to manipulate with multiple auxiliary variables, the stationarity hypothesis is too simple and constructing scenarios can be very time consuming.
In contrast, a stereotypical view sees machine learning as strong when there is a significant amount of data to train with. Deep Learning approaches, which are well established for classification are less developed when used in regression type problems with few training data, the terrain of the Geostatistical model. Problems for the Deep Learning approach so far are the lack of appropriate training data, lack of associated RF and hence uncertainty management.
A class of models that have proved useful in mapping, and increasingly popular, are those based on random forests (or similar). Many published variants of this are only poorly spatially aware. This presentation will show how one approach, developed originally for 3d geological modelling in the oil industry, leverages geostatistical models. It has a simple, yet sufficiently rigorous, statistical interpretation to allow construction of realizations to study the behaviour of the target variable. Using ideas of Structural Equation Models and Interventions from Causal modelling, it is easy to intervene on a realization to emphasise particular features (e.g seismic features or user interpretations). This parametric route easily, and rapidly, allows a wider set of ‘scenarios’ than standard geostatistical models.
Marie Colette Van Lieshout↑
Marie-Colette van Lieshout was educated at Free University and CWI
Amsterdam. She started her career as lecturer at the University of
Warwick before moving back to CWI as senior researcher. She also
holds a chair in spatial stochastics at the University of Twente.
She has published around 60 scientific papers and 5 books in
stochastic geometry, spatial statistics and image analysis. Her
research concerns the modelling and analysis of complicated
geometrical structures such as point and object processes, random
fields and tessellation models. Van Lieshout is an elected member
of the ISI (International Statistical Institute).
She is a board member of the Dutch Royal Mathematics
Society, currently serving as its secretary, a member of the council
of the Bernoulli Society, and was a member of the editorial boards
of several journals, including Bernoulli and
Methodology and Computing in Applied Probability.
Talk title: Parametric and non-parametric monitoring of induced seismicity in the Groningen gas field
Increasing production volumes and the resulting depletion of the gas field have led to induced earthquakes in the previously tectonically inactive Northern Netherlands. The most significant event to date, which occurred in August 2012 near Huizinge with a magnitude of 3.6, attracted massive public attention, prompting the Ministry of Economic Affairs to reduce production volumes.
In this talk, I will discuss parametric and non-parametric techniques for monotoring the induced seismicity with specific attention to the relation between seismic hazard and production volumes as well as pore pressure measurements.
This talk is partially based on joint work with Zhuldyzay Baki.
IAMG Awardees↑
John Carranza - William Christian Krumbein Medal 2022↑
E.J.M. (John) Carranza started his career as exploration geologist/geochemist (1983–2001) in the Bureau of Mines & Geosciences of the Philippines. He was researcher (2001–2003) and then Associate Professor (2003–2012) at the University of Twente (The Netherlands). He was Associate Professor (2013–2016) at the James Cook University (Australia). He was Visiting Professor (2015–2017) at the State University of Campinas (Brazil). He was Professor of Geological Sciences (2017–2021) at the University of KwaZulu–Natal (South Africa). He is currently Professor of Economic Geology at the University of the Free State (South Africa). His fields of expertise are
- geochemistry for mineral exploration, ore genesis and geo-environmental studies,
- spatial mathematics/statistics for predictive modeling of mineral resources and geological hazards, and
- remote sensing for geological/mineral exploration.
Talk title: Multi-scale Spatial Patterns of Mineral Deposits: Their Exploration Significance
Philippe Renard - John Cedric Griffiths Teaching Award 2022↑
Dr. Philippe Renard is Associate Professor of Hydrogeology at the University of Neuchatel Switzerland where he leads the Stochastic Hydrogeology Group. He graduated from the Nancy School of Geology in Nancy, France and obtained his PhD from Paris School of Mines in 1996. His research focuses on stochastic hydrogeology and aquifer. In geostatistics, he has developed multiple- point statistics methods and their applications to a wide range of problems from 3-D geological modeling to the simulation of climate variables. Renard has been the editor of Hydrogeology Journal, president of the geoENVia association and manages the Hydrogeologist Time Capsule.
Talk title: Multiple-Point statistics for stochastic modeling of aquifers, where do we stand?
In the last 20 years, multiple-point statistics have been a focus of much research, successes and disappointments. The aim of this geostatistical approach is to integrate geological information into stochastic models of aquifer heterogeneity to better represent the connectivity of high or low permeability structures in the underground.
Many different algorithms have been and are still proposed. They are all based on the concept of a training data set from which spatial statistics are derived and used in a further step to generate stochastic conditional realizations. While the method clearly succeeded in enabling modelers to generate realistic models, several issues are still the topic of debate both from a practical and theoretical point of view, and some issues such as training data set availability are often hindering the application of the method in practical situations.
In this talk, the aim is to present a review of the status of these approaches both from a theoretical and practical point of view using several examples at different scales and in various geological environment (from pore network to regional aquifer, and from karst to alluvial systems).
Qiuming Cheng - Distinguished Lecturer 2022 ↑
Professor Qiuming Cheng is an internationally recognized leader in mathematical geosciences. His pioneering research on the new fractal density theory and nonlinear mathematical models for singularity analysis made major impacts on several geoscientific disciplines, including those concerned with extreme geological events originated from nonlinear processes of plate tectonics such as formation of supercontinents, magmatism, mid-ocean ridge heat flow, earthquakes and mineralization. His multifractal filtering and singularity analysis techniques are now routinely applied for anomaly identification in mineral exploration and environmental assessment.
Professor Cheng has received several prestigious awards including the William Christian Krumbein Medal, the highest award bestowed by the International Association for Mathematical Geosciences (2008), the National Science and Technology Progress Award awarded by the Chinese Government (2013), and the AAG Gold Medal, the highest award bestowed by the International Association of Applied Geochemists (AAG) (2021). Professor Cheng served as associate editor for Computers & Geosciences and the Journal of Exploration Geochemistry. Professor Cheng co-chaired two IAMG Annual Conferences (Toronto, 2005, Beijing, 2007). He has served as President of the International Association for Mathematical Geosciences (2012-2016), President of the International Union of Geological Sciences (IUGS) (2016-2020) and member of the Committee of Science Planning of International Science Council (ISC) (2019-). Professor Cheng was elected a Member of Chinese Academy of Sciences (CAS) (2019) and a foreign member of Academia European (MAE) (2020). He is now a professor at the School of Earth Sciences and Engineering, Sun Yat-sen University.
Talk title: Quantitative simulation and prediction of extreme geological events
The systematic study of extreme geological events (such as plate collision and subduction, extreme cold and extreme hot events, biological extinction and revival, earthquakes, volcanoes, mineralization, and oil accumulation) that occurred during the evolution of the earth is essential not only for understanding the “abrupt" changes in the evolution of the earth, but also for an in-depth investigation of the co-evolution of material-life-environment of the livable earth. However, due to the temporal and spatial anomalies and complexity of extreme geological events, classical mathematical models cannot be effectively applied to quantitively describe such events. Comparative studies of many types of geological events suggest that such extreme geological events often depict “singular” characteristics (abnormal accumulation of matter or massive release of energy in a small space or time interval). On this basis, the author proposes a unified definition of extreme geological events, a new concept of “fractal density” and a “local singularity analysis” method for quantitative description and modeling of extreme geological events. Applications of these methods to several types of extreme geological events are introduced to demonstrate that the singularity theory and methods recently developed can be used as general approaches for the characterization, simulation, and prediction of extreme geological events.
IAMG2022 sponsored by:
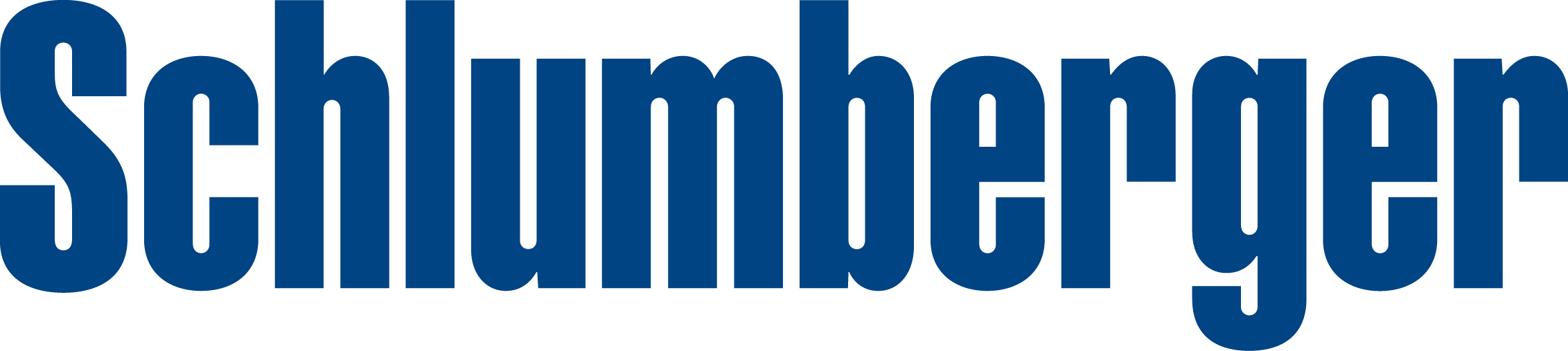
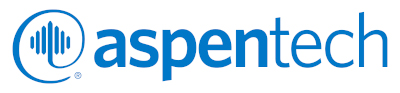
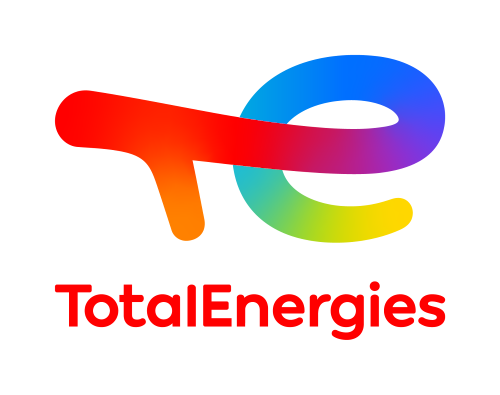
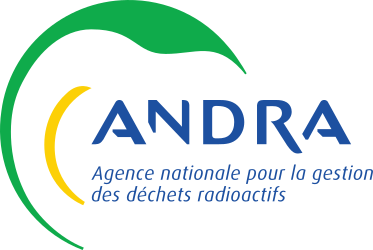